Computer vision, data labeling and annotation, cloud AI services, and intelligence applications are the most mature applications of AI, according to Gartner’s 2023 AI Hype Cycle.

Generative AI and foundation models have reached the Peak of Inflated Expectations in Gartner’s 2023 AI Hype Cycle, which is a global report on the maturity of technologies throughout their life cycles. The Peak of Inflated Expectations is the place for innovations, which have both a lot of success stories and a lot of failures. Some companies act on innovations during the Peak of Inflated Expectations, but most don’t.
Jump to:
Inflated expectations are a normal part of the hype cycle
Generative AI and foundation models may be overhyped; there is more excitement around them than there are use cases, Gartner said. However, the Peak of Inflated Expectations is a normal part of the life cycle of how innovations are brought into the mainstream (Figure A).
Figure A
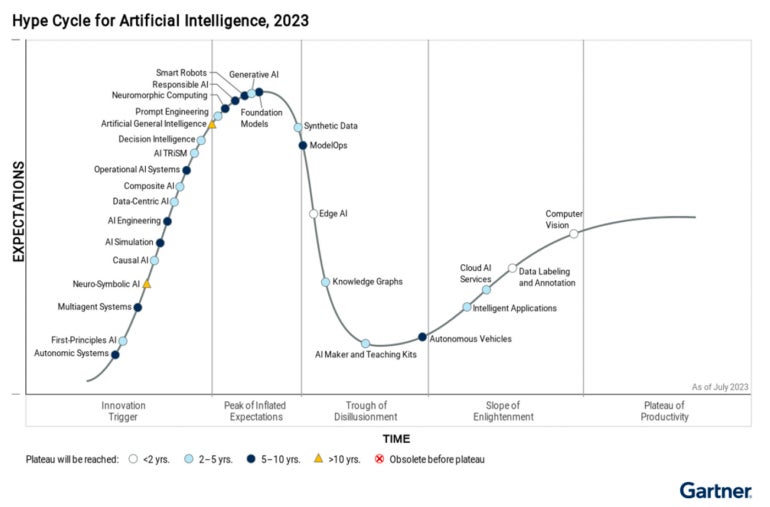
Other notable AI applications are on the peak as well. Smart robots, responsible AI and neuromorphic computing, which uses spiking neural networks instead of deep neural networks to try to replicate the function of a biological brain, are reaching the peak of the hype cycle. That means they’re poised to enter the Trough of Disillusionment, where expectations and investment are cooled before some companies settle on a truly practical and normalized use of the innovation.
Computer vision, data labeling and annotation, cloud AI services and intelligence applications are the most mature technologies in the AI group. Gartner has placed these AI technologies on the Slope of Enlightenment, meaning second and third generations of products have emerged with some bugs worked out, and only more conservative companies remain cautious.
No AI technology has yet reached the Hype Cycle’s Plateau of Productivity, which is the point at which innovation has entered the mainstream and investments have consistently paid off. The hype cycle is meant to demonstrate whether technology buyers should take a risky, moderate or cautious approach to emerging innovations.
SEE: Gartner provided an in-depth look at generative AI in its recent Emerging Technologies Hype Cycle. (TechRepublic)
Some AI technologies have practical business benefits
Gartner found that AI is likely to have some benefit to businesses. Most Hype Cycles have a few emerging technologies that end up being rated low or moderately beneficial; all of the technologies in the AI Hype Cycle were rated high or transformative. The benefit rating ranks how much of a positive impact the innovation could have across industries.
Many of the generative AI-driven technologies in the report need to be combined in order to create practical services, Gartner noted. Data and analytics leaders would be well-served to consider investing first in innovations that have been packaged together as business solutions, such as computer vision, knowledge graphs, smart robots, intelligent applications and AI cloud services. Gartner recommended that data and analytics leaders focus on products that do not require team members to have extensive, specialized engineering or data science skills.
“The focus on generative AI at the moment means that some techniques that will fuel generative AI advancement are receiving more attention now than in previous years,” said report author Afraz Jaffri, director analyst at Gartner.
SEE: Salesforce suggests companies should take practical steps to reduce bias from generative AI. (TechRepublic)
Gartner predicts generative AI and decision intelligence, which involve teaching predictive AI how to affect predicted outcomes, will reach mainstream adoption in two to five years.
“Early adoption of these innovations (generative AI and decision intelligence) will lead to significant competitive advantage and ease the problems associated with utilizing AI models within business processes,” the firm wrote.
Other AI technologies are still searching for use cases
Gartner’s survey shows businesses are becoming disillusioned about ModelOps, edge AI, knowledge graphs, AI maker and teaching kits, and autonomous vehicles. Knowledge graphs, which are machine-readable representations of material assets and how they relate to each other, are moving exceptionally rapidly along the Hype Cycle.
If this rapid movement and the disillusionment seem contradictory, that’s because the Hype Cycle isn’t an ascent from obscure to mainstream. Instead, the Trough of Disillusionment is a low point before technologies enter the upward Slope of Enlightenment.
Knowledge graphs can complement many other AI innovations, such as machine learning, generative AI, search algorithms, smart assistants and recommendation engines.
SEE: Hiring kit: Prompt engineer (TechRepublic Premium)
Up-and-coming AI innovations
The AI innovations that are lowest down in the Innovation Trigger section of the Hype Cycle, meaning they are the least mature, are autonomic or self-managing systems, first-principles or physics-informed AI, multiagent systems and neuro-symbolic AI.
Gartner defines neuro-symbolic AI as a combination of machine learning and symbolic systems such as knowledge graphs in order to give an AI system a more contextual understanding of concepts and reduce hallucinations. Neuro-symbolic AI is estimated to require more than 10 years before it reaches mainstream adoption.
What to ask before investing in generative AI
According to Jaffri, questions that data and analytics leaders should ask themselves before investing in generative AI include:
- How will the performance, accuracy and associated business value of the application(s) be measured?
- What is the acceptable threshold of accuracy that can be tolerated?
- What is the best approach to deployment? Consider choosing between APIs, fine-tuning or retrieval-augmented generation.
- Is there an off-the-shelf solution that can be used to test the benefits of an AI innovation without having to build a home-grown solution?
- How can other AI techniques besides generative AI be leveraged to provide business benefits?
- What assessment framework will you use to determine security and data protection risks?
Source of Article