Tiny aerial drones have many potential uses, but their ability to navigate is severely limited by their minuscule amount of onboard processing power. Scientists have now set about addressing that limitation, taking a cue from foraging insects such as ants.
Among other things, micro drones could one day be performing tasks such as searching for survivors at disaster sites, performing reconnaissance in hazardous environments, or even pollinating crops. In almost all cases, they will be required to autonomously fly out into a specific area, then return to their home base.
When it comes to getting back to base, navigation options currently include GPS when outdoors, or wireless communication with wayfinding-beacon modules when indoors. That said, GPS doesn’t work in indoor environments, and wayfinding modules aren’t likely to be preinstalled in most buildings.
Larger drones may utilize LiDAR and computer vision systems to create 3D maps of their surroundings on their way out, which they subsequently follow to make their way back. Creating such maps requires a lot of processing power and memory, however, which the tiny microprocessors in micro drones simply can’t provide.
One previously proposed alternative involves getting such drones to simply take a series of snapshot photos of their surroundings on their way out. On their way back – assuming they follow the same route – they just seek out the landmarks in those snapshots, in the reverse order that they were taken. While this is a more efficient method of navigation, the number of snapshots required still requires too much memory.
In order to drastically reduce that number, scientists at The Netherlands’ Delft University of Technology (TU Delft) looked to ants and other foraging insects. Ants essentially take mental snapshots as they head out from their colony, but they also (roughly) count the number of steps they take between those snapshots.
This step-counting process, known as odometry, allows them to get away with taking much fewer snapshots than would otherwise be required. They just match their surroundings to one snapshot, take the memorized number of steps, then check the next snapshot. That procedure is repeated snapshot after snapshot, until the insect reaches its colony.
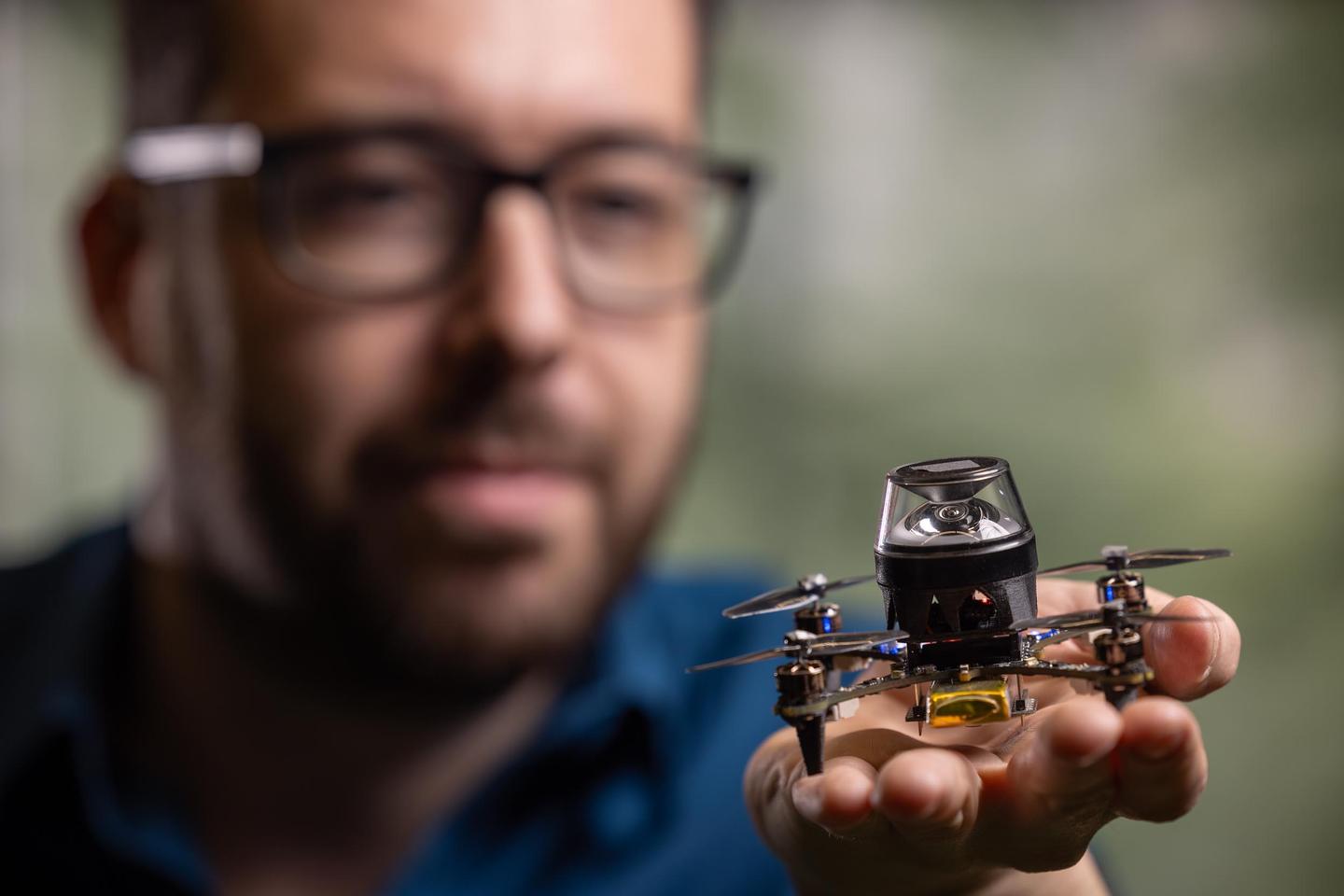
TU Delft
Led by professors Tom van Dijk and Guido de Croon, the TU Delft team applied this same principle to a 56-gram (2-oz) CrazyFlie miniature quadcopter which they equipped with an omnidirectional camera. Of course, aerial drones don’t walk, so the copter can’t count its steps like an ant.
“For odometry, our drone does something similar to honeybees, it integrates the motion determined from optical flow,” de Croon tells us. “For this, our robot has a small downward-pointing camera that tracks how quickly things pass by in the visual field.”
What’s more, that camera also tracks the direction in which the ground passes beneath it.
On its return trip, once the drone has determined that it has travelled the recorded distance/direction from one recorded snapshot, it compares its current camera image to the next recorded snapshot. Given the fact that the aircraft will inevitably have drifted a bit on its way back, it corrects its course until the two images almost exactly match.
“Suppose that there is a tree in sight and it is larger in the snapshot image than the current image. Then the drone needs to move towards that tree, as it will then become bigger in the image as well,” explains de Croon.
Navigating in this fashion within an indoor environment, the drone was able to autonomously make its way back to base along a winding 100-meter (328-ft) obstacle course using just 1.16 kilobytes of memory – that’s well within the capacity of most commercial micro drones. In fact, the quadcopter reportedly now holds the record for being the lightest drone to ever perform vision-based navigation.
You can see it in action, in the following video. A paper on the research was recently published in the journal Science Robotics.
Visual Route-following for Tiny Autonomous Robots – Science Robotics
Source: TU Delft
Source of Article