Data quality refers to the reliability, accuracy, consistency and validity of your data. Measuring data quality ensures that your data is trustworthy and fit for its intended use, whether that’s for analysis, decision-making or other purposes.
High-quality data is dependable, error-free and reflects the true state of your work processes, while low-quality data may be outdated and contain inaccuracies that lead to unreliable results and conclusions.
Ensuring good data quality is crucial for organizations to derive meaningful insights, make informed decisions and maintain peak operational efficiency. Various techniques and processes, such as data cleansing, validation and quality assurance, are employed to improve and maintain superior data quality.
What is the meaning of data quality?
The meaning of data quality is the overall level of completeness and relevance of data for its intended purpose. In simpler terms, data quality describes how good or trustworthy your data actually is. Remember that high-quality data is relevant to the task at hand, while low-quality data may contain errors, which leads to poor analyses.
Data quality is critical across various businesses, where decisions are often based on data-driven insights. Ensuring high data quality involves processes such as data collection and data maintenance to enhance the accuracy and utility of your data over time.
Data quality is essential for organizations and individuals to trust the data they use for routine analysis and a multitude of other use cases.
Data quality vs. data integrity
There tends to be some divide when it comes to defining data quality and data integrity and understanding the nuances and differences between the two. Certain working professionals and organizations sometimes consider data quality and data integrity interchangeable due to their shared similarities and how they often complement each other.
In fact, some treat data quality as a component of data integrity and vice versa, while others view data quality and data integrity as part of a much larger effort to assist with data governance.
Data integrity can also be looked at more broadly, where a multi-faceted effort to ensure accuracy and data security becomes paramount. Data integrity can also prevent data from being configured by unauthorized individuals, where data quality is more generally known for creating a means of achieving specified purposes.
How to measure data quality
Measuring data quality often involves assessing various attributes of datasets to determine their accuracy, completeness, consistency, timeliness and integrity:
- Evaluate the degree to which the data reflects the truth by comparing it against known sources or standards and identifying discrepancies or errors.
- Assess whether the data contains all the necessary information required for its intended purpose by checking for missing values, fields or records that could affect the comprehensiveness of the dataset.
- Analyze the data to ensure that it is internally consistent across different elements or attributes.
- Consider the currency of the data and assess whether it’s up to date for the intended use case.
- Determine the extent to which the data is pertinent and useful for its intended purpose or analysis — this step is very crucial. Assess whether the data provides meaningful insights or answers specific questions, and then evaluate the ease with which users can access and use that data.
Ultimately, measuring data quality involves using a combination of quantitative metrics, assessments and domain knowledge to evaluate each of these attributes. Tools and techniques such as data profiling, data cleansing and data validation can be employed to improve data quality as well.
Examples of data quality metrics
Data quality metrics provide measurable values that indicate how well your data meets specific standards of quality. Examples of these metrics include, but are not limited to, accuracy, completeness and consistency. These metrics matter because they directly impact your organization’s ability to make informed decisions, operate efficiently and maintain trust with stakeholders.
Accuracy refers to how correctly data reflects the real-world entities or values it is supposed to represent. When data is accurate, you can rely on it to make decisions that are based on true and precise information.
Completeness measures whether all necessary data is present. Incomplete data can lead to gaps in information, making it difficult to draw accurate conclusions or take appropriate actions. For instance, if customer records are missing critical details like contact information, it becomes challenging to contact them for marketing or support purposes.
Consistency evaluates whether data is uniform across different datasets and systems. Inconsistent data can create confusion and lead to errors in reporting and analysis.
These metrics matter because they collectively ensure your data is fit for its intended purposes. High-quality data enhances trust, supports effective decision-making and improves operational efficiency. By regularly measuring and maintaining these data quality metrics, your organization can avoid the pitfalls of poor data quality, such as errors, inefficiencies and misinformed decisions.
Popular data quality tools
Popular tools that can best help with data quality include solutions like Talend, Informatica and Trifacta.
These available data quality tools are excellent for assisting with data quality and offer a wide range of options that are sure to satisfy most of your software requirements.
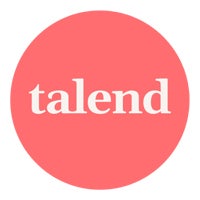
Talend
Talend offers a comprehensive suite for data integration and data integrity, providing robust capabilities for data profiling, cleansing and enrichment. Its open-source nature allows for extensive customization, making it a favorite among organizations looking for flexible and scalable data quality solutions.
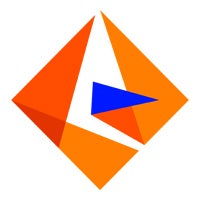
Informatica
Informatica is another leading tool known for its user-friendliness, powerful data quality and data governance features. It provides a range of functionalities, including data profiling, cleansing, matching and monitoring. Informatica’s suite is designed to handle complex data environments, offering advanced algorithms for data integration, data validation and enrichment.
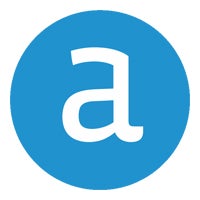
Trifacta
Trifacta focuses on data preparation, offering intuitive and interactive tools for data wrangling. It is designed to streamline the process of cleaning and structuring raw data, making it easier for analysts and data scientists to work with high-quality information. Trifacta’s machine learning capabilities assist in identifying data patterns, suggesting transformations and automating repetitive tasks, which significantly reduce the time and effort needed for data preparation.
Should your organization use data quality?
Using data quality in your organization is crucial because it underpins virtually every aspect of your operations and strategic initiatives. High-quality data ensures the information guiding your decisions is accurate, reliable and comprehensive.
Data quality is also essential for compliance and risk management. Many industries face strict regulatory requirements, and high-quality data ensures you meet these standards, avoiding potential fines as well as legal issues. It also supports accurate reporting and auditing processes, further safeguarding your organization.
Lastly, prioritizing data quality empowers your organization with the tools to operate more efficiently, make better decisions, enhance customer satisfaction and, ultimately, achieve sustained growth and innovation over time.
Frequently asked questions (FAQs)
What are the four elements of data quality?
The four elements of data quality are accuracy, completeness, consistency and timeliness. Accuracy ensures data correctly represents real-world values, completeness ensures all necessary data is present, consistency ensures data is uniform across systems and timeliness ensures data is up to date.
Why is data quality so important?
Data quality is crucial because it ensures accurate, reliable and comprehensive information, which is essential for effective decision-making, operational efficiency and maintaining customer trust.
What is the most significant part of data quality?
The most significant part of data quality is ensuring accuracy, as it guarantees that the data truly represents real-world scenarios and supports reliable decision-making. Without accuracy, all other aspects of data quality, such as completeness and consistency, become less valuable since the foundational data itself is flawed.
Source of Article