Handing the platform run by Databricks to the vendor-neutral foundation will speed growth, the organizations say.
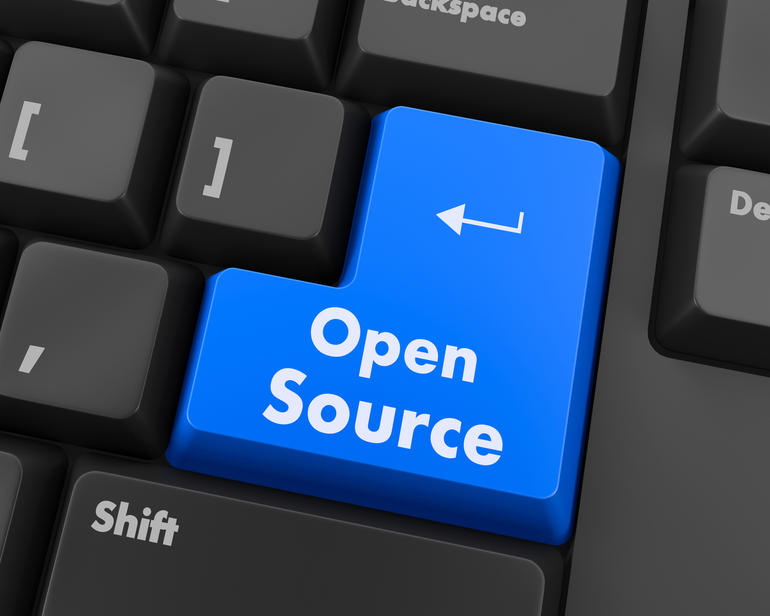
Image: boygovideo, Getty Images/iStockphoto
Databricks, the company behind open source end-to-end machine learning (ML) platform MLflow, announced Thursday that it is handing control of MLflow to the Linux Foundation.
“Our experience in working with the largest open source projects in the world shows that an open governance model allows for faster innovation and adoption through broad industry contribution and consensus building,” said VP of strategic programs at the Linux Foundation Michael Dolan.
Under the control of the foundation, MLflow will be managed using Apache License v.2, which Databricks CEO Ali Ghodsi said will easily allow businesses to use it without worry.
“Handing MLflow over to the Linux Foundation makes it more independent, and will drive even more businesses to contribute to the growth of the platform,” Ghodsi said.
SEE: Hiring Kit: Computer Research Scientist (TechRepublic Premium)
Databricks, which was co-founded by Apache Spark creator Matei Zaharia, released the alpha build of MLflow in 2018, and said it has seen explosive growth in interest and use since then. To contrast, Ghodsi said, it took three years to get the same amount of participation in Spark that MLflow garnered in three months.
MLflow has been adopted for ML data projects by numerous large organizations, such as Microsoft, Accenture, Zillow, Virgin, and Starbucks.
MLflow was built with an open interface “designed to work with any ML library, algorithm, deployment tool or language,” Databricks said in its 2018 MLflow introductory post. Because it’s designed to be end-to-end, MLflow also incorporates every step in the machine learning process from data preparation to presentation of results.
SEE: Robotic process automation: A cheat sheet (free PDF) (TechRepublic)
In the same introductory post, Ghodsi explained that MLflow was designed to address several problems in the machine learning process that Databricks had repeatedly heard mentioned:
- Too many ML products meant wasting time searching for the right combination of tools,
- There are too many variables in each ML experiment to keep track of,
- Reproducibility is difficult because of the above two reasons, and the problem of passing projects between teams working from different perspectives, and
- Deployment of ML models is difficult due to a lack of standardization between tools.
“MLflow keeps this process from becoming overwhelming by providing a platform to manage the end-to-end ML development lifecycle from data preparation to production deployment, including experiment tracking, packaging code into reproducible runs, and model sharing and collaboration,” Databricks said in a press release.
Developers interested in experimenting with MLflow, which is designed to scale from small projects to enterprise-level initiatives, can find out how to install it and learn to use it at MLflow’s GitHub page.
Also see
Source of Article